Data analysis and modelling of cognitive tasks
During my PhD at Aix-Marseille University, I studied the neuronal activity during delayed motor preparation and decision-making. For this, I trained monkeys to perform cognitive tasks and recorded the neuronal activity in several cortical areas, as well as the animal's behavior. Through this experience, I learned to design and conduct behavioral experiments, perform extracellular recordings in vivo, and analyze and interpret neuropsychological data. This project resulted in several articles (Ponce-Alvarez et al. 2010; Kilavik et al. 2009, 2010, 2012; Confais et al. 2012) that jointly showed that the motor cortex activity exhibits context-dependency and processing of perceptual cues relevant for movements. In parallel, I investigated the temporal evolution of decision-making processes in sensory and frontal cortices of monkeys performing a perceptual discrimination task. Using a machine-learning approach, based on hidden Markov models (Ponce-Alvarez et al. 2012)—a method successfully applied in later studies (e.g. Tatsuno et al. 2020)—, we showed that the decision is reliably maintained in frontal lobes by a cascade of transient metastable events. More recently, I developed a neural network model to study how amygdala neurons implement sequential decision-making to efficiently solve decision problems involving multiple objects (Grabenhorst et al. 2023).
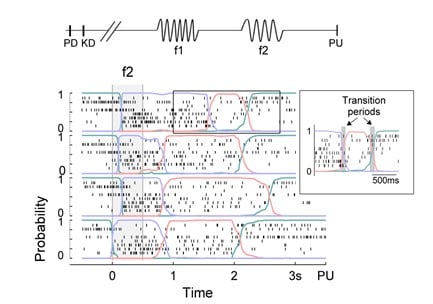
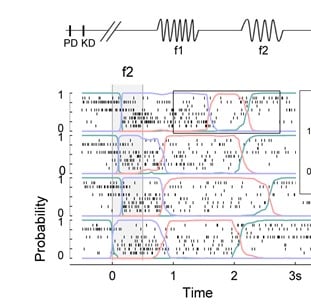
Sequences of metastable states during decision-making. Top: Discrimination task. Monkeys compared the frequency of two mechanical vibrations, f1 and f2, sequentially delivered to their fingertip and report their perceptual evaluation (whether f1<f2, or not) by pressing one of two buttons. KD: trial initiation; PU: response signal. Bottom: Hidden Markov Models (HMMs) describe the neuronal ensemble spiking activity in single trials as a sequence of firing rate states. Here, the spiking activity of an example ensemble of neurons in the primary motor cortex (M1) is presented together with the likelihood of each HMM state (colored traces) for four trials, during the decision period. We showed that frontal lobes display decision-selective sequences of states with sharp transitions occurring during the stimulus presentation and the delay period with high trial-by-trial variability. State sequences in neuronal ensembles of frontal lobes were predictive of the monkey’s decision, with 62-100% accuracy. Figure adapted from Ponce-Alvarez et al. (2012, J Neurosci).